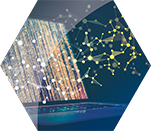
Cambridge Healthtech Institute’s Inaugural
AI/ML for Early Drug Discovery
Improving Speed and Efficiency for Target Discovery, Drug Design, and Optimisation
4 - 5 DECEMBER 2024
Cambridge Healthtech Institute’s conference on Artificial Intelligence (AI)/Machine Learning (ML) for Early Drug Discovery offers case studies presented by experts in academia and industry showing how and where AI/ML has been integrated and implemented in drug discovery. Chemists, biologists, pharmacologists, and bioinformaticians will highlight how AI predictions, ML algorithms, and data science can help identify and validate drug targets, design and prioritise lead candidates for development, and enable data-driven decision-making.